Transformer in PyTorch
PyTorchは、Attention is All You Needを基に実装された、標準的なTransformerのモジュールが用意されている。
nn.Transformerモジュールは、単一でも使用しやすいように、高度にモジュール化されており、簡単に、nn.Transformerモジュールをコンポーネントに適用したり、あるいはnn.Transformerモジュールを使って、コンポーネントを作成したりすることができる。
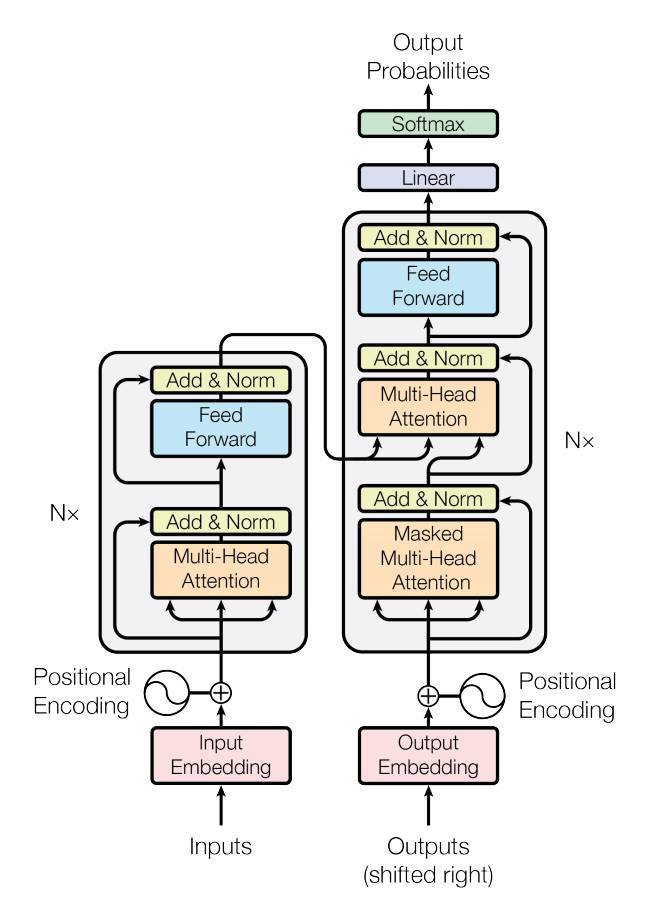
Model Architecture
class TransformerModel(nn.Module):
def __init__(self, ntoken, ninp, nhead, nhid, nlayers, dropout=0.5):
super(TransformerModel, self).__init__()
from torch.nn import TransformerEncoder, TransformerEncoderLayer
self.model_type = 'Transformer'
self.src_mask = None
self.pos_encoder = PositionalEncoding(ninp, dropout)
encoder_layers = TransformerEncoderLayer(ninp, nhead, nhid, dropout)
self.transformer_encoder = TransformerEncoder(encoder_layers, nlayers)
self.encoder = nn.Embedding(ntoken, ninp)
self.ninp = ninp
self.decoder = nn.Linear(ninp, ntoken)
self.softmax = nn.LogSoftmax(dim=-1)
self.init_weights()
def _generate_square_subsequent_mask(self, sz):
mask = (torch.triu(torch.ones(sz, sz)) == 1).transpose(0, 1)
mask = mask.float().masked_fill(mask == 0, float('-inf')).masked_fill(mask == 1, float(0.0))
return mask
def init_weights(self):
initrange = 0.1
self.encoder.weight.data.uniform_(-initrange, initrange)
self.decoder.bias.data.zero_()
self.decoder.weight.data.uniform_(-initrange, initrange)
def forward(self, src):
if self.src_mask is None or self.src_mask.size(0) != src.size(0):
device = src.device
mask = self._generate_square_subsequent_mask(src.size(0)).to(device)
self.src_mask = mask
src = self.encoder(src) * math.sqrt(self.ninp)
src = self.pos_encoder(src)
output = self.transformer_encoder(src, self.src_mask)
output = self.decoder(output)
output = self.softmax(output)
return output
Positional Encoding
class PositionalEncoding(nn.Module):
def __init__(self, d_model, dropout=0.1, max_len=5000):
super(PositionalEncoding, self).__init__()
self.dropout = nn.Dropout(p=dropout)
pe = torch.zeros(max_len, d_model)
position = torch.arange(0, max_len, dtype=torch.float).unsqueeze(1)
div_term = torch.exp(torch.arange(0, d_model, 2).float() * (-math.log(10000.0) / d_model))
pe[:, 0::2] = torch.sin(position * div_term)
pe[:, 1::2] = torch.cos(position * div_term)
pe = pe.unsqueeze(0).transpose(0, 1)
self.register_buffer('pe', pe)
def forward(self, x):
x = x + self.pe[:x.size(0), :]
return self.dropout(x)
パラメータは固定されていて、可視化することができる。
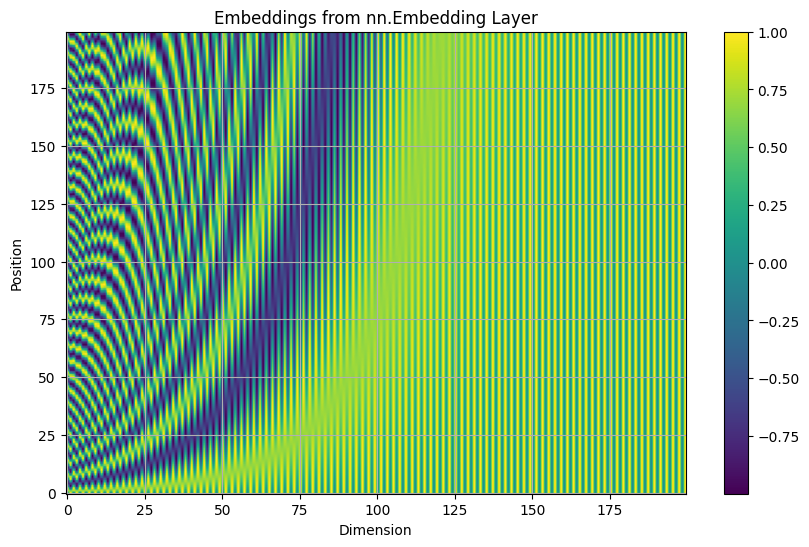
Data Perparation
import torchtext
from torchtext.data.utils import get_tokenizer
TEXT = torchtext.data.Field(tokenize=get_tokenizer("basic_english"),
init_token='<sos>',
eos_token='<eos>',
lower=True)
train_txt, val_txt, test_txt = torchtext.datasets.WikiText2.splits(TEXT)
TEXT.build_vocab(train_txt)
device = torch.device("cuda" if torch.cuda.is_available() else "cpu")
def batchify(data, bsz):
data = TEXT.numericalize([data.examples[0].text])
# データセットをbszサイズに分割した際のバッチ数を求める
nbatch = data.size(0) // bsz
# Trim off any extra elements that wouldn't cleanly fit (remainders).
data = data.narrow(0, 0, nbatch * bsz)
# Evenly divide the data across the bsz batches.
data = data.view(bsz, -1).t().contiguous()
return data.to(device)
batch_size = 20
eval_batch_size = 10
train_data = batchify(train_txt, batch_size)
val_data = batchify(val_txt, eval_batch_size)
test_data = batchify(test_txt, eval_batch_size)
Get Batch
batchifyメソッドで、入力データをミニバッチに分割する。
グループの数は引数のbszで指定。
bsz(バッチサイズ)を4とした場合
入力
出力
Make Training Data
get_batchメソッドを使って、学習用データ(または検証用データ)と正解データを作成する。
正解データは、学習用データの単語列を、1単語前にずらして単語列を作成する。
先程のアルファベットのミニバッチを入力とし、iを0、bpttを2とした場合、get_batchの出力は以下のようになる
(左・学習用データ、右・訓練用データ)。
bptt = 35
def get_batch(source, i):
seq_len = min(bptt, len(source) - 1 - i)
data = source[i:i+seq_len]
target = source[i+1:i+1+seq_len].view(-1)
return data, target
Masking
generate_square_subsequent_maskを使って、Transformerが学習を行う際に、現在注目している単語に後続する単語を参照できなくする。
def _generate_square_subsequent_mask(self, sz):
mask = (torch.triu(torch.ones(sz, sz)) == 1).transpose(0, 1)
mask = mask.float().masked_fill(mask == 0, float('-inf')).masked_fill(mask == 1, float(0.0))
return mask
Initiate Model
ntokens = len(TEXT.vocab.stoi) # the size of vocabulary
emsize = 200 # embedding dimension
nhid = 200 # the dimension of the feedforward network model in nn.TransformerEncoder
nlayers = 2 # the number of nn.TransformerEncoderLayer in nn.TransformerEncoder
nhead = 2 # the number of heads in the multihead attention models
dropout = 0.2 # the dropout value
model = TransformerModel(ntokens, emsize, nhead, nhid, nlayers, dropout).to(device)
Run the Model
criterion = nn.CrossEntropyLoss()
lr = 5.0 # 学習率
optimizer = torch.optim.SGD(model.parameters(), lr=lr)
scheduler = torch.optim.lr_scheduler.StepLR(optimizer, 1.0, gamma=0.95)
import time
def train():
model.train()
total_loss = 0.
start_time = time.time()
ntokens = len(TEXT.vocab.stoi)
for batch, i in enumerate(range(0, train_data.size(0) - 1, bptt)):
data, targets = get_batch(train_data, i)
optimizer.zero_grad()
output = model(data)
loss = criterion(output.view(-1, ntokens), targets)
loss.backward()
torch.nn.utils.clip_grad_norm_(model.parameters(), 0.5)
optimizer.step()
total_loss += loss.item()
log_interval = 200
if batch % log_interval == 0 and batch > 0:
cur_loss = total_loss / log_interval
elapsed = time.time() - start_time
print('| epoch {:3d} | {:5d}/{:5d} batches | '
'lr {:02.2f} | ms/batch {:5.2f} | '
'loss {:5.2f} | ppl {:8.2f}'.format(
epoch, batch, len(train_data) // bptt, scheduler.get_lr()[0],
elapsed * 1000 / log_interval,
cur_loss, math.exp(cur_loss)))
total_loss = 0
start_time = time.time()
def evaluate(eval_model, data_source):
eval_model.eval()
total_loss = 0.
ntokens = len(TEXT.vocab.stoi)
with torch.no_grad():
for i in range(0, data_source.size(0) - 1, bptt):
data, targets = get_batch(data_source, i)
output = eval_model(data)
output_flat = output.view(-1, ntokens)
total_loss += len(data) * criterion(output_flat, targets).item()
return total_loss / (len(data_source) - 1)
Try the Model
def generate(model, prompt, max_len=50, temperature=1.0):
model.eval()
predicted = prompt.cuda()
with torch.no_grad():
for i in range(max_len):
output = model(predicted)
word_weights = output[-1].squeeze().div(temperature).exp().cpu()
word_idx = torch.multinomial(word_weights, 1).cuda()
predicted = torch.cat((predicted, word_idx.unsqueeze(0)), dim=0)
return predicted
prompt_str = "i am a "
tokenizer = get_tokenizer('basic_english')
prompt_tokens = tokenizer(prompt_str)
prompt = torch.tensor([TEXT.vocab.stoi[token] for token in prompt_tokens]).unsqueeze(1).to(device)
predicted = generate(best_model, prompt, max_len=50, temperature=0.9)
predicted_str = ' '.join([TEXT.vocab.itos[idx] for idx in predicted])
print(predicted_str)
Appendix
学習前のEmbedding
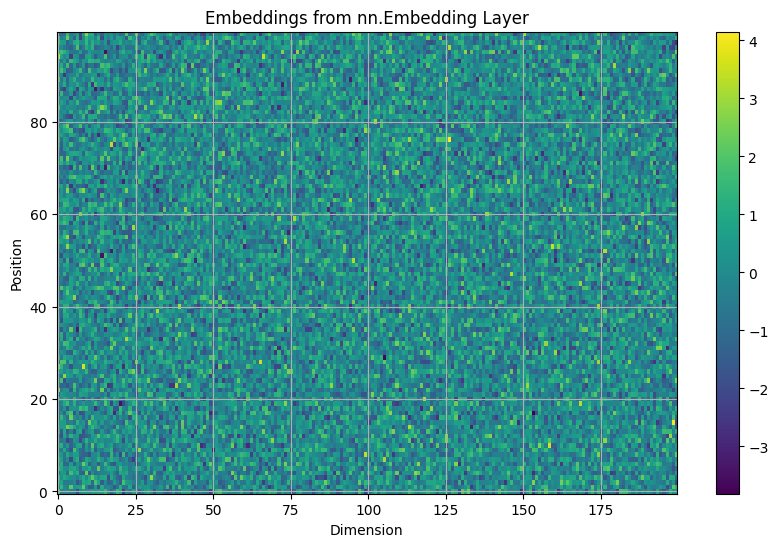
学習後のEmbedding
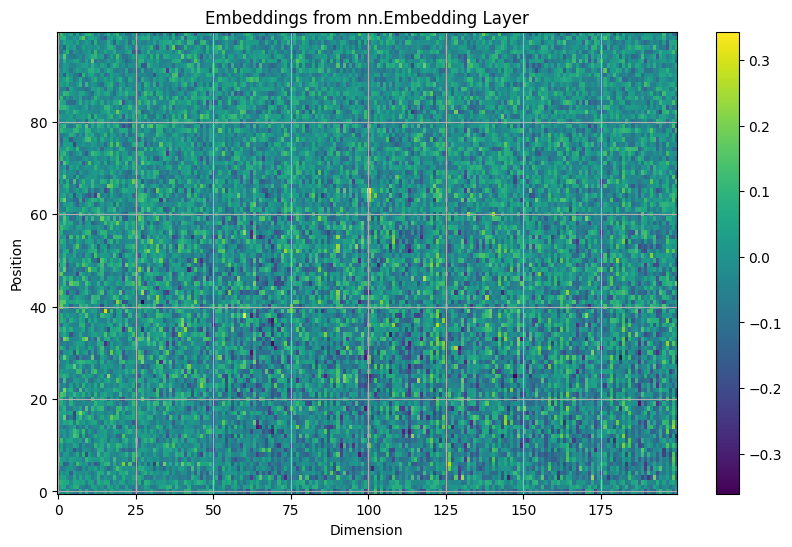
Comment